本文使用LeNet5识别手写数字。
LetNet-5 的基本结构
LeNet-5包含7层网络结构(不含输入层),包含两个卷积层、两个降采样层(池化层)、两个全连接层和输出层。
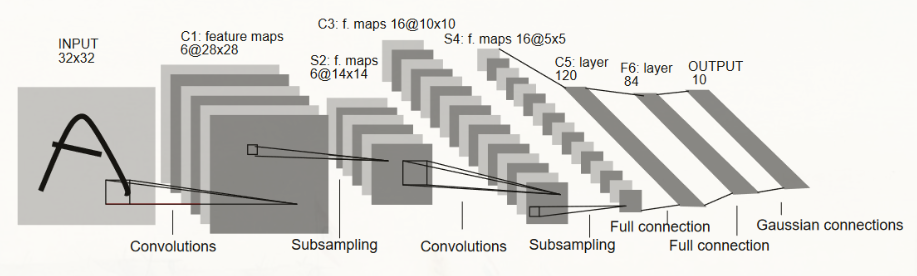
输入层的大小为32×32手写图像,在实际应用中,通常会对图像进行预处理,如对像素进行归一化。
2、卷积层C1(Convolutional layer
C1)
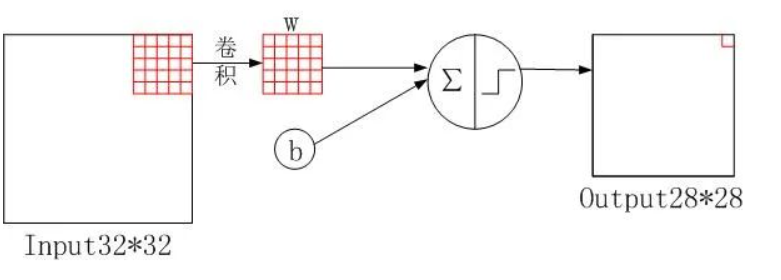
代码:
1
| nn.Conv2d(1, 6, kernel_size=5, padding=2)
|
根据代码的含义,通道数从1变6,原图的尺寸从32×32变为28×28,这与卷积核大小、步幅和\(padding\)有关,其输出的特征图像尺寸如下:
\[
output size = \frac{W-kernelsize+2×padding}{stride}+1
\] 其中,\(W\)表示输入图像的宽度。 \[
output size = \frac{32-5+2×0}{1}+1 = 28
\] 代码:
在卷积操作完成之后会进行归一化(Batch
Normalization)操作提高神经网络和加速收敛
3、采样层S2(Subsampling layer
S2)
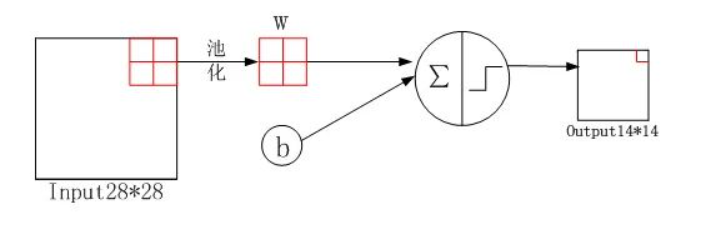
代码:
1
| nn.Conv2d(6, 16, kernel_size=5)
|
通道数没有发生过改变,计算公式如下: \[
outputsize =\frac{W-poolsize}{stride}+1 = \frac{28-2}{2}+1 = 14
\] 然后同上,进行批量归一化。
4、卷积层C3(Convolutional layer
C3)
代码:
1
| nn.Conv2d(6, 16, kernel_size=5)
|
通道数由6变16,输出特征图尺寸为10x10,具体计算如下: \[
output size = \frac{W-kernelsize+2×padding}{stride}+1 =
\frac{14-5+2×0}{1}+1 = 10
\] 该层较为特殊,16 个卷积核并不是都与 S2 的 6
个通道层进行卷积操作,如下图所示,C3 的前六个特征图(0,1,2,3,4,5)由 S2
的相邻三个特征图作为输入,对应的卷积核尺寸为:5x5x3;接下来的 6
个特征图(6,7,8,9,10,11)由 S2
的相邻四个特征图作为输入对应的卷积核尺寸为:5x5x4;接下来的 3
个特征图(12,13,14)号特征图由 S2
间断的四个特征图作为输入对应的卷积核尺寸为:5x5x4;最后的 15 号特征图由
S2 全部(6 个)特征图作为输入,对应的卷积核尺寸为:5x5x6。
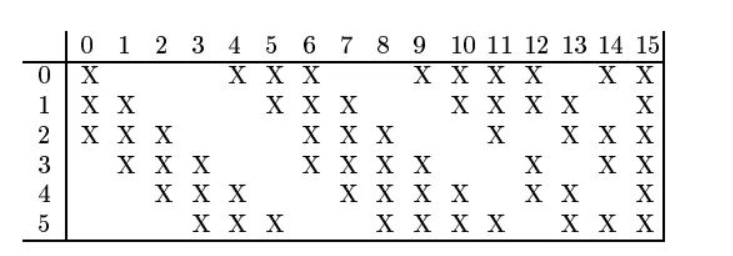
5、采样层S4(Subsampling layer
S4)
代码:
1
| nn.AvgPool2d(kernel_size=2, stride=2),
|
特征图尺寸由10×10变成5×5,输出通道还是16。 \[
outputsize = \frac{W-kernelsize}{stride}+1 = \frac{10-2}{2} +1 = 5
\]
6、全连接层C5(Fully
connected layer C5)
代码:
1
| nn.Linear(16 * 5 * 5, 120)
|
C5将每个大小为 5×5
的特征图拉成一个长度为400(维度为1X16X5X5)的向量,并通过一个带有120个神经元的全连接层进行连接。120是由LeNet-5的设计者根据实验得到的最佳值。
7、全连接层F6(Fully
connected layer F6)
全连接层F6将120个神经元连接到84个神经元。
8、输出层(Output layer)
输出层由10个神经元组成,每个神经元对应0-9中的一个数字,并输出最终的分类结果。
搭建网络
1 2 3 4 5 6 7 8 9 10 11 12 13
| import torch from torch import nn from d2l import torch as d2l
net = nn.Sequential( nn.Conv2d(1, 6, kernel_size=5, padding=2), nn.Sigmoid(), nn.AvgPool2d(kernel_size=2, stride=2), nn.Conv2d(6, 16, kernel_size=5), nn.Sigmoid(), nn.AvgPool2d(kernel_size=2, stride=2), nn.Flatten(), nn.Linear(16 * 5 * 5, 120), nn.Sigmoid(), nn.Linear(120, 84), nn.Sigmoid(), nn.Linear(84, 10))
|
加载数据集
1 2 3 4 5 6 7 8 9 10 11 12 13 14 15 16 17 18 19 20 21 22 23 24 25 26 27 28 29 30
| train_dataset = torchvision.datasets.MNIST(root = './data', train = True, transform = transforms.Compose([ transforms.Resize((32,32)), transforms.ToTensor(), transforms.Normalize(mean = (0.1307,), std = (0.3081,))]), download = True)
test_dataset = torchvision.datasets.MNIST(root = './data', train = False, transform = transforms.Compose([ transforms.Resize((32,32)), transforms.ToTensor(), transforms.Normalize(mean = (0.1325,), std = (0.3105,))]), download=True)
batch_size = 64
train_loader = torch.utils.data.DataLoader(dataset = train_dataset, batch_size = batch_size, shuffle = True)
test_loader = torch.utils.data.DataLoader(dataset = test_dataset, batch_size = batch_size, shuffle = False)
|
- 测试阶段的
shuffle=False
:在测试阶段,通常不需要打乱数据的顺序。测试时模型是在未见过的数据上进行评估,因此希望模型看到的是原始数据的有序顺序,以便能够更好地评估模型的泛化性能。如果在测试时也打乱数据,可能会导致模型在评估时看到的数据分布与实际场景不一致。(其实如果是True
影响也不大)
设置评价指标
1 2 3 4 5 6 7 8 9 10 11 12 13 14 15 16 17 18
| def evaluate_accuracy_gpu(net, data_iter, device=None): """使用GPU计算模型在数据集上的精度""" if isinstance(net, nn.Module): net.eval() if not device: device = next(iter(net.parameters())).device metric = d2l.Accumulator(2) with torch.no_grad(): for X, y in data_iter: if isinstance(X, list): X = [x.to(device) for x in X] else: X = X.to(device) y = y.to(device) metric.add(d2l.accuracy(net(X), y), y.numel()) return metric[0] / metric[1]
|
模型训练
1 2 3 4 5 6 7 8 9 10 11 12 13 14 15 16 17 18 19 20 21 22 23 24 25 26 27 28 29 30 31 32 33 34 35 36 37 38 39 40
| def train_ch6(net, train_iter, test_iter, num_epochs, lr, device): """用GPU训练模型(在第六章定义)""" def init_weights(m): if type(m) == nn.Linear or type(m) == nn.Conv2d: nn.init.xavier_uniform_(m.weight) net.apply(init_weights) print('training on', device) net.to(device) optimizer = torch.optim.SGD(net.parameters(), lr=lr) loss = nn.CrossEntropyLoss() animator = d2l.Animator(xlabel='epoch', xlim=[1, num_epochs], legend=['train loss', 'train acc', 'test acc']) timer, num_batches = d2l.Timer(), len(train_iter) for epoch in range(num_epochs): metric = d2l.Accumulator(3) net.train() for i, (X, y) in enumerate(train_iter): timer.start() optimizer.zero_grad() X, y = X.to(device), y.to(device) y_hat = net(X) l = loss(y_hat, y) l.backward() optimizer.step() with torch.no_grad(): metric.add(l * X.shape[0], d2l.accuracy(y_hat, y), X.shape[0]) timer.stop() train_l = metric[0] / metric[2] train_acc = metric[1] / metric[2] if (i + 1) % (num_batches // 5) == 0 or i == num_batches - 1: animator.add(epoch + (i + 1) / num_batches, (train_l, train_acc, None)) test_acc = evaluate_accuracy_gpu(net, test_iter) animator.add(epoch + 1, (None, None, test_acc)) print(f'loss {train_l:.3f}, train acc {train_acc:.3f}, ' f'test acc {test_acc:.3f}') print(f'{metric[2] * num_epochs / timer.sum():.1f} examples/sec ' f'on {str(device)}')
|
animator = d2l.Animator(xlabel='epoch', xlim=[1, num_epochs],legend=['train loss', 'train acc', 'test acc'])
此代码为绘制折线图
- 以上代码个 epoch
结束时都会计算并得到一次测试集的准确率,并将其记录下来
最终得到训练结果
1 2
| lr, num_epochs = 0.9, 10 train_ch6(net, train_iter, test_iter, num_epochs, lr, d2l.try_gpu())
|
1 2
| loss 0.469, train acc 0.823, test acc 0.779 55296.6 examples/sec on cuda:0
|